Where there’s smoke, there’s AI
In 2022, wildfires affected over 7.5 million acres across the U.S prompting new collaborations between edge solution providers like AMAX, and AI/ML researchers to develop new AI powered wildfire detection and prevention systems. These systems are designed to monitor, detect, and respond to wildfires in rapidly accelerated timeframes.
Historically, fire prevention relied on fire lookouts stationed in towers across mountain tops, who would report smoke and potential fires by telephone, carrier pigeon, or heliograph.
Today, fire detection systems look a little different. Utilizing 360° cameras and space satellites equipped with advanced algorithms, these devices detect wildfires at their point of origin. Live feeds from these cameras are monitored by AI and alerts are sent to experts in command centers who then dispatch response teams upon confirmation of the data.
The backbone of this technological evolution are AI and Deep Learning algorithms, trained on hundreds of thousands of hours of video data of smoke and fire tracking for enhanced detection and analysis.
These algorithms run on extremely specialized hardware, including high-density clusters for training and weatherproof edge solutions for inferencing.
Let’s look at how several hardware and software developers are working together to combat wildfires globally.
NVIDIA and Lockheed Martin’s Wildfire Prediction
In a major initiative to address the increasing severity of wildfires, NVIDIA has partnered with Lockheed Martin, alongside state and federal agencies, to deploy AI and digital twin simulation technologies to predict and manage future wildfires. Their collaborative effort includes establishing the world’s first AI-centric lab dedicated to wildfire prediction and response, harnessing NVIDIA’s AI infrastructure and visualization platform, Omniverse. The lab is set to be a hub for innovation, bringing together industry experts and resources to develop the Cognitive Mission Manager (CMM) system, which integrates real-time data from various sources to predict fire spread and provide actionable insights for firefighting efforts.
The lab’s location in Silicon Valley will provide a collaborative space for the rapid development of AI-driven wildfire management solutions. Lockheed Martin contributes its expertise in AI and data science, while NVIDIA brings to the table its data analysis and simulation capabilities, using systems like the DGX H100 and Omniverse Enterprise to crunch vast amounts of data for visualizing and predicting fire spread. Omniverse’s capability to create digital twins of environments allows for the analysis of fire behavior, comparison of predictions from AI models and the established Rothermel fire spread model, and evaluation of suppression strategies, further illustrating the merging of AI with traditional fire modeling techniques.
DigitalPath’s Real-Time Fire Detection Technology
In a similar project, DigitalPath’s AI system, as part of the ALERTCalifornia initiative, is driving an innovative effort utilizing AI to combat wildfires. This system, powered by accelerated GPUs and networking systems and supported by a vast camera network deployed state-wide, has been designed to detect fires in real-time and provide crucial alerts to first responders.
DigitalPath’s AI, which began operating across CAL FIRE’s command centers in 2023, processes millions of images daily to detect and alert authorities to wildfires, demonstrating how AI can scale and enhance human capabilities in disaster response scenarios. The success of this system has inspired talks of expanding this technology to detect a broader range of natural disasters and replicate its effectiveness across the Western United States through DigitalPath’s ALERTWest subsidiary.
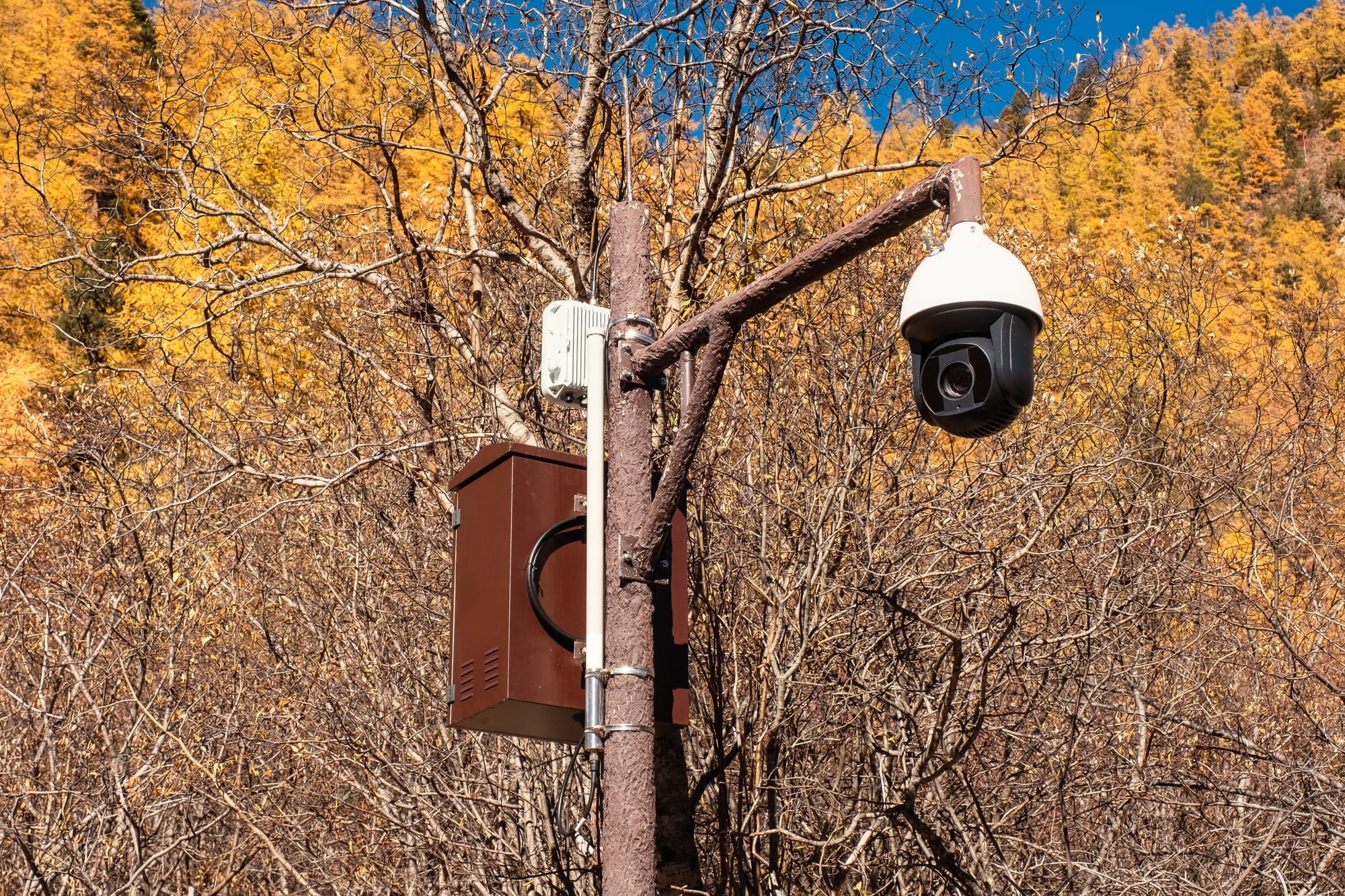
These efforts embody the intersection of technology and environmental stewardship, with AI becoming an instrumental tool in managing and mitigating the impacts of wildfires, which have been a persistent threat, particularly in regions like California.
Expanding the Horizon with Multiple AI Approaches
Efforts to combat wildfires have seen a significant boost with the integration of AI technologies. PanoAI, a San Francisco-based startup, is at the forefront with its Pano Rapid Detect platform. This platform is designed to accelerate the detection and response times for wildfires. The early moments of a fire are critical, and PanoAI’s system empowers detection specialists with advanced visual tools and AI-driven data analysis to quickly identify and contain fires, which is crucial in preventing small flare-ups from becoming raging wildfires.
Pano Stations, strategically positioned on high vantage points, employ 360-degree, ultra-high-definition cameras coupled with AI to monitor and signal wildfire activity across a wide area. The system’s deep learning AI and computer vision automatically detect, verify, and classify wildfire events, delivering real-time alerts and detailed imagery to fire monitoring professionals.
This rapid confirmation and dissemination of information, enabled by PanoAI’s innovative use of technology, offers a significant advantage, allowing responders to act with speed and precision to protect lives, property, and forests.
Space-Based Wildfire Detection with OroraTech and NASA’s FIRMS
OroraTech is another company specializing in the early detection and monitoring of wildfires, however, they are doing so from space, equipping users with the ability to detect hotspots and monitor areas susceptible to wildfires.
By integrating data from more than twenty satellite sources with cutting-edge wildfire algorithms, OroraTech provides a comprehensive service for asset protection, offering users crucial information for early wildfire detection through their Wildfire Solution Pro Pilot Program, which utilizes the FOREST-2 satellite data.
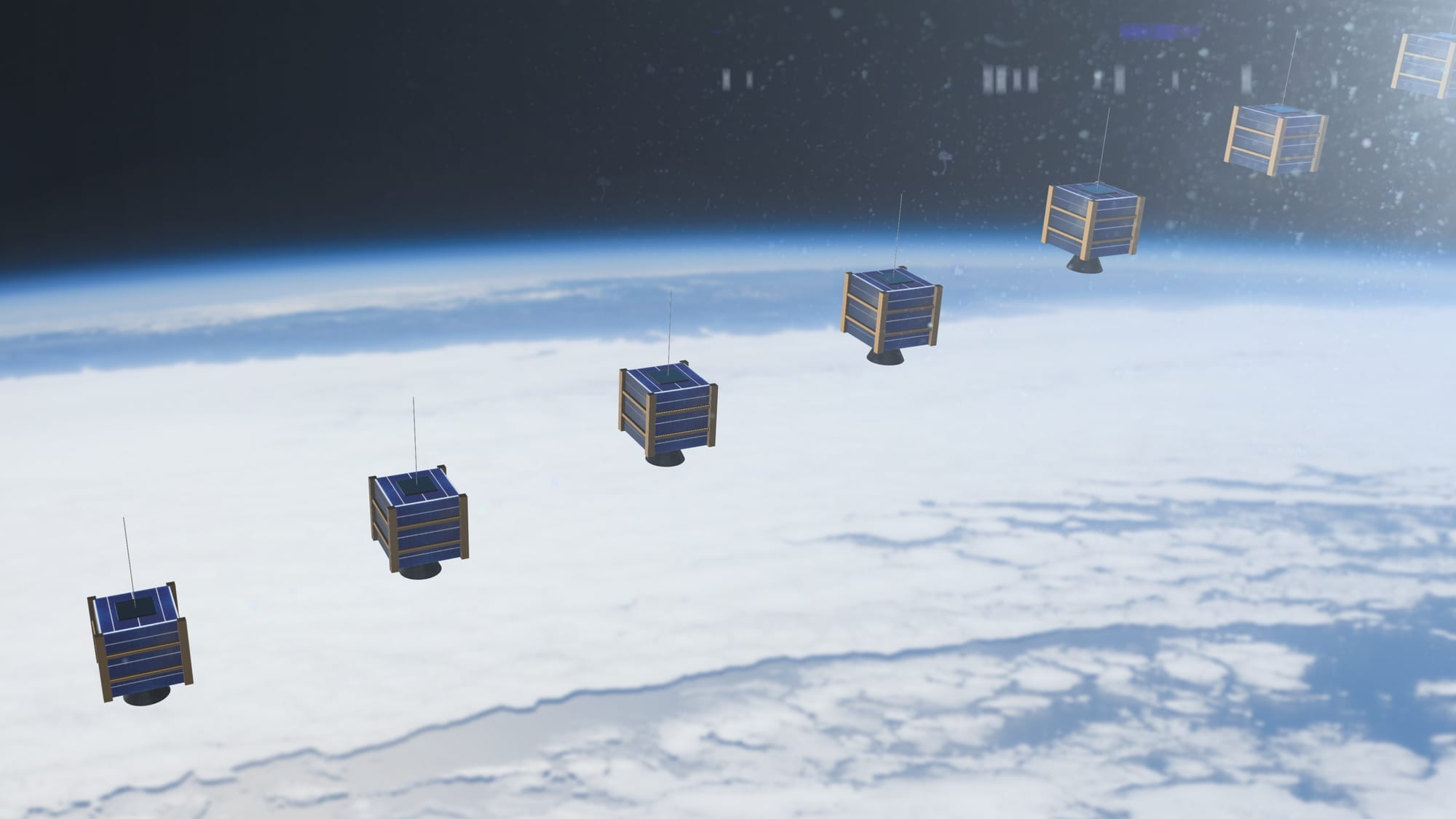
Another space detection system currently being developed is NASA’s Fire Information for Resource Management System (FIRMS). This global fire map has revolutionized the detection of wildfires by integrating ultra-real-time (URT) data from MODIS and VIIRS instruments on NASA and NOAA satellites. This service, a first for the continental United States, boasts a remarkable detection latency of less than 60 seconds from the moment of satellite observation to the identification of a wildfire.
Enabled by the Space Science and Engineering Center at the University of Wisconsin-Madison and funded by NASA’s WildFireSense program, the system streams data directly from ground-based antennas to a central hub where it is processed in real-time. The URT data is currently received from multiple locations including Madison, Wisconsin; Hampton, Virginia; and Mayaguez, Puerto Rico, with expansions planned for additional ground stations across the United States.
The processed URT and Real-Time (RT) fire detections are made available through FIRMS and FIRMS US/Canada Fire maps, identifiable by the URT or RT suffix in the version information. These detections are also integrated into map services and can be viewed in near real-time by users who can track satellite overpasses and observe fires within minutes of their detection. This system not only ensures rapid dissemination of wildfire information but also maintains data consistency with the Near Real-Time (NRT) data from the LANCE FIRMS active fire algorithms, providing a seamless transition as newer data becomes available.
Fire Risk Assessment with Terrafuse
Microsoft’s Terrafuse is deploying advanced artificial intelligence models on the Microsoft Azure platform to enhance the granularity of wildfire risk assessment.
This effort integrates data from previous wildfire events, real-time satellite data, and computational simulations to construct predictive models that deliver hyperlocal risk analysis. These models, informed by physical properties and historical patterns, provide a robust framework for forecasting wildfire threats.
To disseminate this information, Terrafuse offers API access and sophisticated graphical interfaces that facilitate the interpretation of fire risk projections. This approach allows stakeholders such as governmental agencies, insurance firms, and the public to make informed decisions by evaluating potential fire hazards in specific locales with a high degree of accuracy.
Essential Hardware for Effective AI Wildfire Detection
The hardware infrastructure required to support AI wildfire detection systems, especially during the training phase, is robust and demands high computational power.
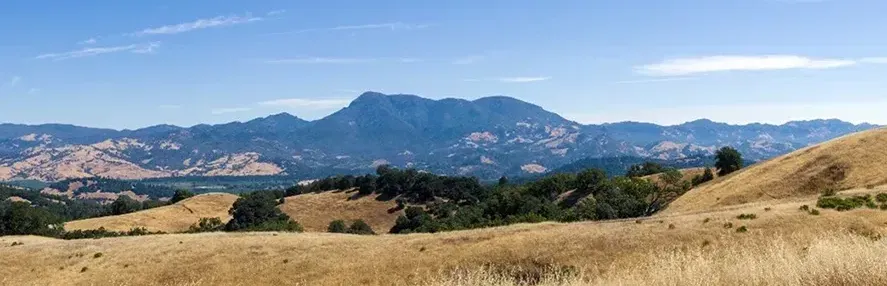
Training complex AI models to accurately detect and predict wildfires involves processing vast datasets, which includes satellite imagery, historical fire data, and real-time environmental sensor data. This requires the use of high-performance GPUs (Graphics Processing Units) and TPUs (Tensor Processing Units), which can handle parallel processing tasks efficiently.
This infrastructure often relies on powerful servers with high-capacity, fast-access storage systems to manage the influx of large-scale data and enable rapid model iteration and development. Cloud computing platforms can also offer scalable overflow solutions that can be crucial for handling excess computational loads and storing the copious amounts of data involved in working with AI models.
At the edge, where real-time fire detection is critical, the hardware is optimized for speed and reliability in harsh environments.
Edge systems typically include a range of IoT devices, such as infrared sensors, cameras equipped with computer vision capabilities, and other environmental monitoring equipment. These devices must be capable of low-latency operation, often running on dedicated edge computing hardware that can process data on-site to provide immediate alerts.
The hardware is also designed to be energy-efficient and rugged, able to withstand the environmental rigors associated with wildfire-prone areas. Solar-powered units with backup battery systems are common to ensure continuous operations.
Connectivity is another critical component, with satellite communication systems often employed to ensure real-time data transmission even from remote locations. These edge systems are crucial for initiating a swift response and providing the first line of data for the AI systems that will continually learn and improve from each event.
AMAX’s Tailored Computing Solutions
AMAX specializes in engineering custom solutions that meet the rigorous demands that are present when designing AI-driven wildfire detection systems, from large-scale data center solutions to edge AI deployments. For large-scale data centers, which are pivotal in training AI models for wildfire detection, that integrate state-of-the-art GPUs and storage technologies. These systems are designed to handle the heavy computational workload and the rapid processing of vast datasets.
AMAX’s computing offerings come with robust storage systems and optimized network setups, providing the necessary efficient data movement and access, which are critical for training AI models and running simulations.
When it comes to edge AI, where immediacy and durability are crucial, AMAX provides specialized computing solutions that can be deployed in remote areas for real-time data processing. These systems are optimized for deployment in remote terrains, providing the backbone for real-time, on-site data handling. Integral to these systems’ design is their support for satellite and telecommunications infrastructure, crucial in the early sensing and methodical monitoring of wildfires.
With customization at the forefront, AMAX’s engineering expertise enables the deployment of AI wherever it’s needed, from the depths of data centers to the front lines of fire detection, ensuring that vital data is captured, analyzed, and acted upon with precision and speed.
A Future Fortified Against Wildfires
The integration of AI in wildfire detection and management represents a significant shift in environmental monitoring and disaster response. With companies like AMAX providing the critical hardware infrastructure, from data center solutions for AI model training to rugged edge computing systems for on-site data processing, the capacity to detect and respond to wildfires is dramatically improving.
This synergy between advanced AI algorithms and custom-engineered hardware is not only enhancing the accuracy and speed of wildfire detection but also empowering firefighting efforts with real-time data and predictive insights, thereby safeguarding natural landscapes, property, and lives with greater efficiency than ever before.